Details
About the talk
A range of zero-permission sensors is found in modern smartphones to enhance user experience. These sensors can lead to unintentional leakage of user private data. We combine leakage from a pool of zero-permission sensors, to reconstruct
Presentation from the talk
Able to classify all 10,000 PIN combinations, results show up to 83.7% success within 20 tries in a single user setting. Firstly, we show that by training on several users, the PIN recovery success can be boosted, when a target user is part of the training pool. On the other hand, PIN recovery is still possible when training pool is mutually exclusive to the target user, albeit with a low success rate.
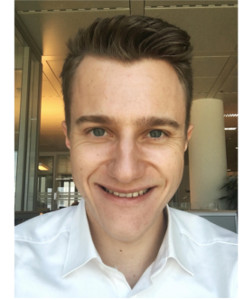
David Berend
Technology Consultant
Recent Comments